
PhD Thesis Defense
March
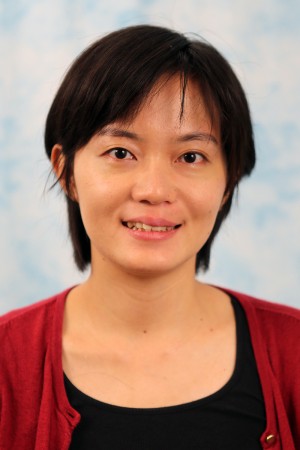
Abstract:
Maps, as our prior understanding of the environment, play an essential role for many modern robotic applications. The design of maps, in fact, is a non-trivial art of balance between storage and richness. In this thesis, we explored map compression for image-to-LiDAR registration, LiDAR-to-LiDAR map registration, and image-to-SfM map registration, and finally, inspired by the promising results of recent NeRF works, we developed a LiDAR-assisted NeRF system that encodes the rich appearance and geometry details of an outdoor environment.
In this talk, we will focus on our most recent work, a LiDAR-assisted Neural Radiance Fields system. Existing NeRF methods usually require specially collected hyper-sampled source views and do not perform well with canonical camera-LiDAR datasets such as autonomous driving datasets. Here we demonstrate that such datasets can be used to construct high quality neural renderings.
Our design leverages 1) LiDAR sensors for strong 3D geometry priors that significantly improve the ray sampling locality, and 2) Conditional Adversarial Networks (cGANs) to recover image details since aggregating embeddings from imperfect LiDAR maps cause artifacts in the synthesized images. The experiments show that while NeRF baselines produce either noisy or blurry results on Argoverse 2, our method produces visually realistic novel view images and outperforms baselines significantly in quantitative metrics. In addition, we explored several applications, including data augmentation, detection simulation, and seasonal change effect. We hope this work to be a step forward to bridge the modern NeRF research and practical applications in real-world outdoor environments.
Thesis Committee Members:
Michael Kaess
Simon Lucey
Matthew Johnson-Roberson
Ian Reid, University of Adelaide