
PhD Thesis Proposal
March
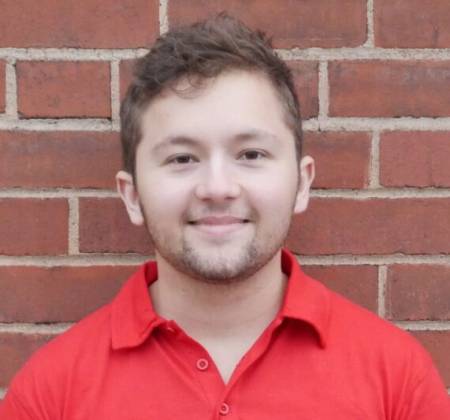
Abstract:
This thesis explores estimating and reasoning about model deviation in robot learning for manipulation to improve data efficiency and reliability to enable real-robot manipulation in a world where models are inaccurate but still useful. Existing strategies are presented for improving planning robustness with low amounts of real-world data by an empirically estimated model precondition to guide a model-based planner or use a model-free skill.
Then, approaches to reduce the amount of real-robot data required to compute reliable plans with inaccurate models are described. The first uses model disagreement to guide exploration. The second addresses how to efficiently collect data to learn a dynamics model and corresponding model precondition. Lastly, the thesis suggests research directions to explore in order to scale model deviation estimates on high-dimensional data.
Thesis Committee Members:
Oliver Kroemer, Chair
Maxim Likhachev
David Held
Dmitry Berenson, University of Michigan