
PhD Thesis Proposal
March
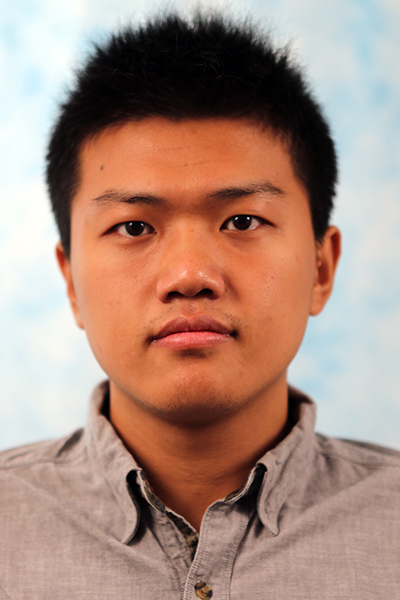
Abstract:
Realistic human avatars play a key role in immersive virtual telepresence. To reach a high level of realism, a human avatar needs to faithfully reflect human appearance. A human avatar should also be drivable and express natural motions. Existing works have made significant progress on building drivable realistic face avatars, but they rarely include realistic dynamic hair which is an important part of human appearance. In pursuit of drivable, realistic human avatars with dynamic hair, we focus on the problem of automatically capturing and animating hair from multi-view videos.
We first look into the problem of capturing the motion of head with near static hair. Because the hair has complex geometry, we use a neural volumetric repre- sentation which can be rendered efficiently and photorealistically. To learn such a representation, we employ an ’analysis-by-synthesis’ strategy that optimizes the representation with the gradient from the reconstruction loss on 2D via differen- tiable volumetric rendering.
Then we extend the problem to capturing hair with dynamics. To accommodate the complexity introduced by the temporal dimension, data-priors on motion like optical flow and point flow are leveraged as additional supervision. To be more specific, we first perform tracking on hair strands with a data prior on motion. In the next step, we attach volumetric primitives to the tracked hair strands to learn the fine level appearance and geometry via differentiable rendering. We further design a differentiable volumetric rendering algorithm with optical flow to ensure temporal smoothness at a fine level.
We then address the problem of building a hair dynamic model for animation. In contrast to the previous two problems that focus on reconstructing 3D/4D, the main difficulty of this problem lies in generating novel animation in 4D. To solve the problem of generation, we present a two-stage pipeline to build a hair dynamic model in a data-driven manner. The first stage performs hair state compression using an autoencoder-as-a-tracker strategy. The second stage learns a hair dynamic model in a supervised manner using the hair state data from the first stage. The hair dynamic model is designed to perform hair state transitions conditioned on head motions and head relative gravity direction.
In the proposed work, we plan to explore rapid capture of personalized hair. One challenge is that multiview capture systems are usually expensive and not readily available for individuals. Another challenge is how to perform fast capture of photorealistic avatars with different hairstyles. To solve those problems, we propose to perform rapid capture of hair for a new subject in-the-wild with a single RGB- D camera by leveraging a 3D hair prior model from capture studio data of other subjects.
Thesis Committee Members:
Jessica Hodgins, Chair
Fernando De La Torre
Jun-Yan Zhu
Michael Zollhoefer, Meta Reality Labs
Kalyan Sunkavalli, Adobe Research