
PhD Thesis Proposal
April
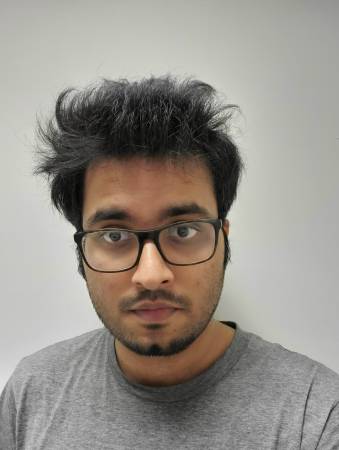
Abstract:
In this thesis, we seek to learn a generalizable goal-conditioned policy that enables zero-shot robot manipulation — interacting with unseen objects in novel scenes without test-time adaptation. Robots that can be reliably deployed out-of-the-box in new scenarios have the potential for helping humans in everyday tasks. Not requiring any test-time training through demonstrations or self-practice before solving a specified task is an important desiderata for the system to be repeatedly usable without any downtime, and safe to work alongside humans without performing any exploratory actions.
Towards this goal, we will investigate how simply scaling robot data and policy architectures is not a feasible way for achieving diverse generalization, and motivate the need to leverage easily available passive web videos for manipulation. In particular, we will first show how we can use pre-trained inpainting models to semantically augment robot interaction data at no additional robot/human cost. This enables generalization to diverse table-top manipulation tasks beyond those seen in the original interaction data. Next, we will demonstrate how we can train a factorized goal-conditioned policy by learning to predict motion trajectories from web videos, and combining that with limited robot interaction data for generalization to unseen object manipulation in-the-wild. For the in-the-wild manipulation experiments, we will show results with a Franka arm on wheels, and with a Spot robot dog equipped with an arm.
In the proposed works, we will discuss and solicit feedback on 1) devising flexible goal-conditioning for zero-shot manipulation such that either a language instruction or an image goal can be input to the policy, and 2) extending goal-conditioned policy learning to long-horizon manipulation by first predicting subgoals and then reliably executing each intermediate task.
Thesis Committee Members:
Abhinav Gupta, Co-chair
Shubham Tulsiani, Co-chair
Oliver Kroemer
Sergey Levine, UC Berkeley