
PhD Speaking Qualifier
May
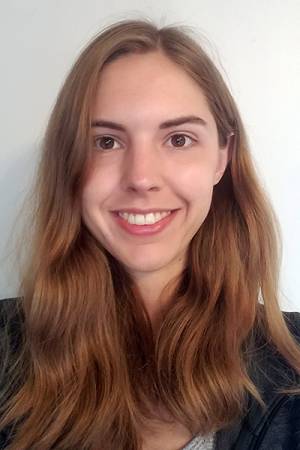
Abstract:
Mobile robots exploring unknown, natural environments with limited communication must map their surroundings using onboard sensors. In this context, terrain mapping can rely on Gaussian process models to incorporate spatial correlations and provide uncertainty estimates when predicting ground height – however, these models fail to account for the oblique viewpoint of a sensor on a ground-based mobile robot. To address this problem, we introduce range-based Gaussian process maps, a local 3D terrain representation capable of modeling range and its associated uncertainty from a LiDAR sensor. Such a model aligns the uncertainty produced from the GP regression with the sensor uncertainty at each observation point. Experimental evaluation on simulated natural terrain indicates that range-based GP maps perform comparably to elevation-based methods at predicting the local terrain height, with the former providing a more accurate representation of the underlying sensor uncertainty. Additionally, range-based GP maps are capable of modeling overhangs and vertical obstacles with ease, which is demonstrated through examples of local range-based GP maps built on real-world data from a fully 3D subterranean environment.
Committee:
David Wettergreen
Michael Kaess
Wennie Tabib
Jason Zhang