
PhD Thesis Proposal
December
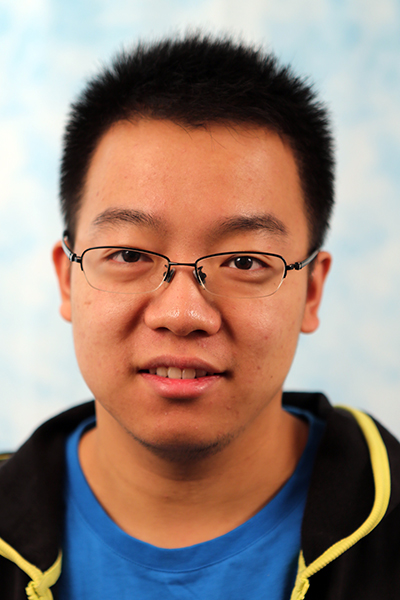
Abstract:
Intelligent robotic agents need to reason about the dynamics of their surrounding world, and use such dynamics reasoning to make future predictions for efficient task planning. In addition, it is also desirable for robots to associate past experience in their memories to their current observation, and conduct analogical reasoning to complete tasks at their hands. In this thesis, we explore both the future prediction and past experience association aspects for learning robotic manipulation policies. Specifically, we first investigate aspects in learning more generalizable dynamics prediction models, where we propose to leverage 3D spatial representations and effective network architectures to learn dynamics models with capabilities of performing spatial reasoning, and adapting efficiently in novel environments. These studies learn neural dynamics models using data collected from existing physics simulators, mainly designed for rigid objects and environments. Afterwards, we extend our problem domain to more complex manipulation settings involving highly deformable objects and fluids, which poses new challenges due to the high dimensional state space and complex underlying dynamics. Such problem setting is still beyond the scope of current robot learning literature, and since there’s no useful simulators available, we develop our own differentiable simulation engine and propose to address the problem via leveraging gradient information from differentiable simulation, and present a comprehensive benchmark environment for evaluating and inspiring future research work in this context. Lastly, we direct our attention from future prediction to past experience association, and propose to perform analogical reasoning using past experience stored in memories for few shot manipulation learning and more efficient task adaptation.
Thesis Committee Members:
Katerina Fragkiadaki, Chair
David Held
Deepak Pathak
Shuran Song, Columbia University
Chuang Gan, MIT-IBM Watson AI Lab