
PhD Thesis Proposal
December
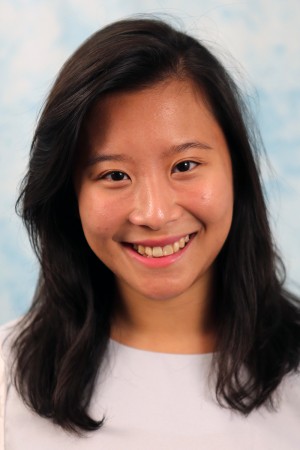
Abstract:
On-road autonomous driving has seen rapid progress in recent years with driverless vehicles being tested in various cities worldwide. However, this progress is limited to cities with well-established infrastructure and has yet to transfer to off-road regimes with unstructured environments and few paved roads. Advances in high-speed and reliable autonomous off-road driving can unlock immense potential in less-accessible areas, increasing productivity in applications such as agriculture, logistics, defense, and humanitarian operations.
Over the past decade, much work has been done in developing autonomous off-road driving agents, however, most are limited to low speed regimes. The key challenge limiting speed is the short sensing range in which the agent can reliably perceive environmental features pertinent to safe and efficient navigation. First, sensing at farther distances is often noisy, and sometimes cues helpful for intelligent long-term planning are beyond sensing range. Second, the agent operates in a wide spectrum of environments that differ greatly in appearance. Finally, occlusions are prevalent, resulting in vast unknown regions.
In this thesis, I seek to address the above challenges to increase the reliable sensing area for safe and efficient off-road driving. First, in ongoing work, I introduce MOVE, a multi-modal voxel mapping framework that uses cameras and LiDAR to increase reliable sensing range to beyond LiDAR range. In the proposed research, I build on the ongoing work to further expand the sensing area. To achieve better performance in new environments, I propose ALTER, an adaptive self-supervised visual semantic framework for accurate long-range predictions under distribution shift. Then, I will explore a self-supervised method to “hallucinate” unknown regions blocked by occlusions. Finally, I would like to evaluate the system-level impacts of the proposed algorithms (e.g. safe speed, number of collisions) across varying scenarios. To this end, I propose an off-road perception benchmark that provides quantitative navigation results on real-world data in a scalable, offline manner.
Thesis Committee Members:
Sebastian Scherer, Chair
Matthew Johnson-Roberson
Deva Ramanan
Ali-akbar Agha-mohammadi, Jet Propulsion Laboratory