
PhD Thesis Proposal
November
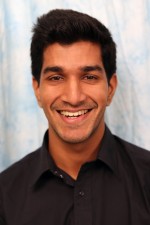
Abstract:
Humans possess a remarkable ability to learn to perform tasks from a variety of different sources-from language, instructions, demonstration, etc. In each case, they are able to easily extract the high-level strategy to solve the task, such as the recipe of cooking a dish, whilst ignoring irrelevant details, such as the precise shape of their cooking pan. Humans can do so by virtue of their ability to reason abstractly about the tasks at hand, and the behaviors needed to solve these tasks.
Inspired by this, this thesis aims to enable robots with the ability to build temporal abstractions over behaviors-human and robots alike-and tasks they seek to solve. This entails answering the following two research questions – (Q1) How can robots learn to acquire and represent behaviors-or skills-that may be composed to solve a variety of tasks? and (Q2) How do robots learn to reason about these skills and their effects to solve complex tasks?
In our background work, we consider the first question, i.e., how agents can go about acquiring and representing new behaviors for themselves. We present two methods to do so, adopting an unsupervised representation learning perspective to the problem.
In completed work, we shift focus to answering the second question, i.e., how agents can reason about the skills they have to solve various tasks. We first focus particularly on the learning from demonstration setting. We explore how agents can learn correspondences between their own skills, and those of a demonstrator, or how we can translate skills from demonstrators to agents, inspired by unsupervised machine translation in natural language.
In proposed work, we begin to consider the effects of agent behaviors on their environments. We first consider how agents can jointly reason about the skills they execute and the effects these skills have on the objects and environments that these agents interact with. We then propose to explore how agents can reason about environment-aware task strategies that they-and demonstrators-use to solve tasks. Particularly, we propose to explore how we can translate more environment-aware task strategies from human demonstrators to robots. We finally discuss future directions that the work in this thesis enables.
Thesis Committee Members:
Jean Oh, Chair
David Held
Shubham Tulsiani
Amy Zhang, UT Austin