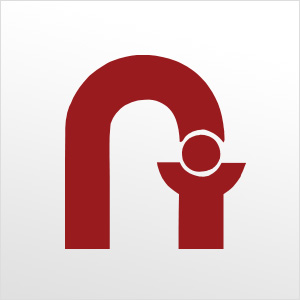
Abstract:
Real-world environments, such as homes, hospitals, and restaurants, often contain many objects that a robot could possibly manipulate. However, for a given manipulation task, only a small number of objects and object properties may actually be relevant. This talk presents CREST (Causal Reasoning for Efficient Structure Transfer), our approach to learn the relevant state space for a robot manipulation policy. CREST conducts interventions using internal models: simulations with approximate dynamics and simplified assumptions that are analogous to human physical intuition. These interventions elicit the structure between the state and action spaces, enabling construction of lightweight neural network policies with only relevant states as input. After pretraining with the internal model, these policies are transferred to a target domain (e.g., the real world) for fine tuning. We perform policy transfer experiments using a high-fidelity robot simulator for two representative manipulation tasks (block stacking and crate opening) in addition to a real robot system for the block stacking task. Our policies are shown to be more robust to domain shifts, more sample efficient to learn, and scale to more complex settings with larger state spaces. Our “structural sim-to-real” approach captures the intuition that it may be easier to first determine which state variables are important using an approximate simulation, and then efficiently fine-tune online to characterize how exactly they matter for solving the manipulation task.
Committee:
Oliver Kroemer, Chair
Chris Atkeson
Jeff Schneider
Brian Okorn