
This event has passed.
VASC Seminar
January
29
Mon
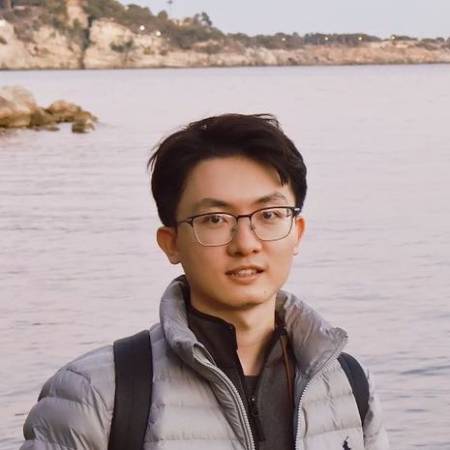
Zhenglun Kong
Ph.D. in the Department of Electrical and Computer Engineering
Northeastern University
Monday, January 29
3:30 pm to 4:30 pm
Newell-Simon Hall 3305
3:30 pm to 4:30 pm
Newell-Simon Hall 3305
Towards Energy-Efficient Techniques and Applications for Universal AI Implementation
Abstract:
The rapid advancement of large-scale language and vision models has significantly propelled the AI domain. We now see AI enriching everyday life in numerous ways – from community and shared virtual reality experiences to autonomous vehicles, healthcare innovations, and accessibility technologies, among others. Central to these developments is the real-time implementation of high-quality deep learning models, facilitated by the proliferation of mobile and embedded computing devices. These advancements have seamlessly integrated machine intelligence into various aspects of our lives. However, the efficient training and inference of Deep Neural Networks, especially in large transformer-based models, remain a major challenge due to high computational demands. This talk will address innovative, efficient deep learning techniques to overcome these hurdles. Zhenglun Kong’s focus on optimizing model performance in a sustainable manner, aligning with the goal of making AI accessible to all. By developing models that are not only powerful but also resource-efficient, we pave the way for AI’s integration into more diverse and resource-constrained environments, democratizing access to these transformative technologies
Bio:
Zhenglun Kong received his B.E. degree in Optoelectronic Information Science and Engineering from Huazhong University of Science and Technology, Wuhan, China. He is currently pursuing his Ph.D. in the Department of Electrical and Computer Engineering at Northeastern University, Boston, U.S., supervised by Professor Yanzhi Wang. He was a research intern at Microsoft Research, ARM, and Samsung Research. His research is primarily focused on the development of efficient deep learning methodologies tailored for real-world scenarios. This includes efficient pre-training/fine-tuning and inference, model/data compression, and efficient DNN design for language (LLMs, BERT) and vision (ViTs, Diffusion) models. He has delved deeply into various CV and NLP tasks and applications, including image/text classification and generation, autonomous driving systems, medical AI (image segmentation, fariness), synthetic data generation, and more. He has published multiple papers in the fields of AI & Machine Learning (NeurIPS, ICML, ECCV, AAAI, CVPR, EMNLP, IJCAI, etc.) and beyond.
Homepage: Zhenglun Kong (zlkong.github.io)
Sponsored in part by: Meta Reality Labs Pittsburgh