
VASC Seminar
February
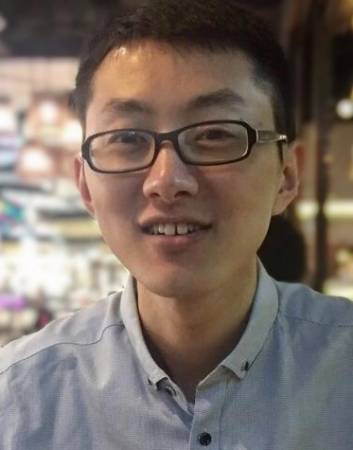
Abstract:
Deep neural networks have achieved great success in various visual applications, when trained with large amounts of labeled in-domain data. However, the networks usually suffer from a heavy performance drop on the data whose distribution is quite different from the training one. Domain adaptation methods aim to deal with such performance gap caused by the domain shift of input. The key to the success of adaptation methods lies in the domain-invariant feature learning. However, most works tend to ignore the specific requirement of downstream tasks, and the resulting features are not discriminative enough. In this talk, I will discuss our recent efforts in learning discriminative domain-invariant features to benefit the downstream tasks. First, I will introduce a method named “Pixel-Level Cycle Association” to facilitate the domain adaptive semantic segmentation. This method takes the abundant pixel-wise relationships into consideration to mitigate the domain shift. Second, I will talk about the Contrastive Adaptation Network, which introduces a class-aware way to learn discriminative features for the cross-domain classification tasks. Finally, I will give a brief summary about this talk.
BIO:
Guoliang Kang is a postdoctoral research associate in Carnegie Mellon University, working with Prof. Alex Hauptmann. He was awarded a PhD degree from the University of Technology Sydney. He is interested in generalizable feature learning under limited supervision. Specifically, his research interests include deep learning, visual domain adaptation, unsupervised learning, etc. More information can be found in https://kgl-prml.github.io/.
Homepage: https://kgl-prml.github.io/
Sponsored in part by: Facebook Reality Labs Pittsburgh