
VASC Seminar
December
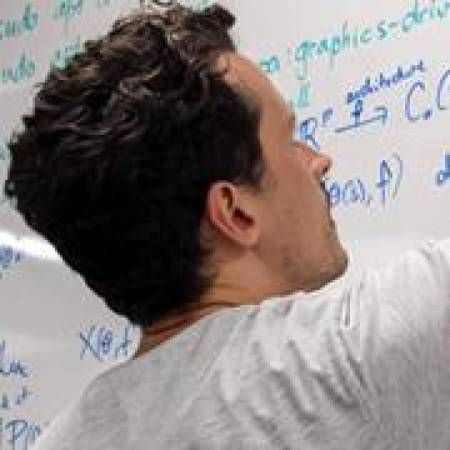
3:00 pm to 4:00 pm
Newell-Simon Hall 3305
Abstract: Precise understanding of the training of deep neural networks is largely restricted to architectures such as MLPs and cost functions such as the square cost, which is insufficient to cover many practical settings. In this talk, I will argue for the necessity of a formal theory of deep optimisation. I will describe such a formal framework, introduced recently by myself and collaborators, which elucidates the roles played by skip connections and normalisation layers in global optimisation and facilitates the first proof that a class of deep nets can be trained to global optima with the cross-entropy cost. I will outline how the theory can be applied even to practical architectures such as ResNet in predicting architectural interventions that accelerate training, on practical datasets such as ImageNet. I will conclude with a discussion of intriguing directions for future research stemming from our work.
Bio: A pure mathematician by training, Lachlan was a pure mathematics postdoc at the Australian National University and the University of Adelaide from late 2019 to mid 2021, when he joined as a postdoc at the Australian Insitute for Machine Learning. Since then, he has been researching equivariance, optimisation and generalisation in deep learning.
Homepage: https://researchers.adelaide.edu.au/profile/lachlan.macdonald
Sponsored in part by: Meta Reality Labs Pittsburgh