
This event has passed.
VASC Seminar
February
5
Mon
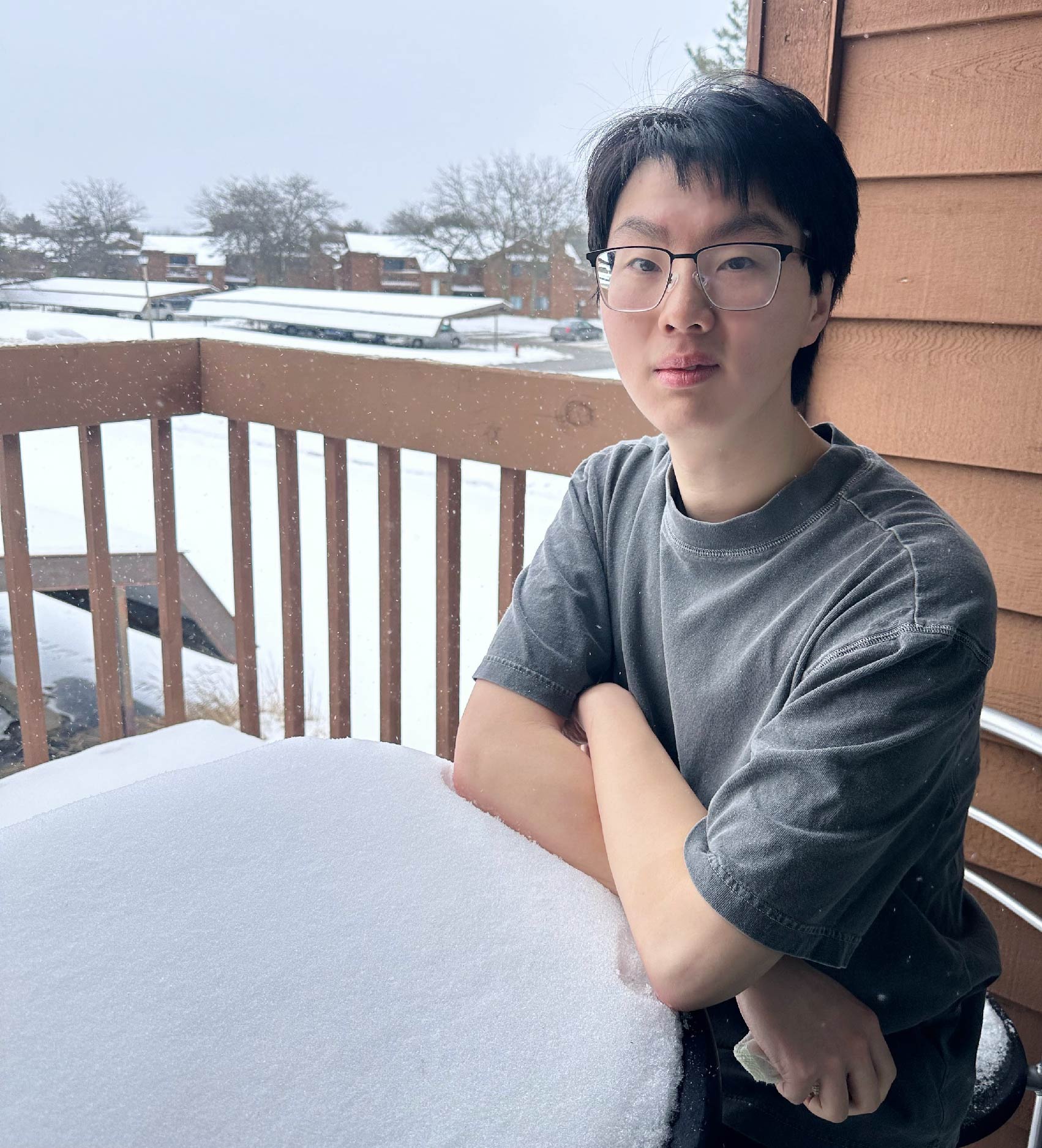
Shengjie Zhu
Ph.D. Student
Michigan State University
Monday, February 5
3:30 pm to 4:30 pm
Newell-Simon Hall 3305
3:30 pm to 4:30 pm
Newell-Simon Hall 3305
Structure-from-Motion Meets Self-supervised Learning
Abstract:
How to teach machine to perceive 3D world from unlabeled videos? We will present new solution via incorporating Structure-from-Motion (SfM) into self-supervised model learning. Given RGB inputs, deep models learn to regress depth and correspondence. With the two inputs, we introduce a camera localization algorithm that searches for certified global optimal poses. However, the optimality is “half-done”. Only poses are optimized, while depth and correspondence remain unchanged. We then leave their fine-tuning as self-supervision with known poses. We introduce a technique that utilizes NeRF to triangulate pseudo-groundtruth for depth and correspondence models. The scheme is initially verified in seconds duration video. On SfM, the method significantly outperforms COLMAP in localization accuracy. On self-supervision, even with seconds duration videos, the algorithm enhances the State-of-The-Art supervised depth and correspondence models.
Bio:
Shengjie Zhu is a final-year Ph.D. student from Michigan State University supervised by Dr.Xiaoming Liu. He obtained his bachelor degree from Southeast University in 2017. His research focuses on multi-view based 3D perception, including depth estimation, correspondence estimation, camera calibration, and camera localization. He aims to scale up 3D perception via learning with unlabeled videos.
Homepage: shngjz.github.io
Sponsored in part by: Meta Reality Labs Pittsburgh