
PhD Thesis Defense
December
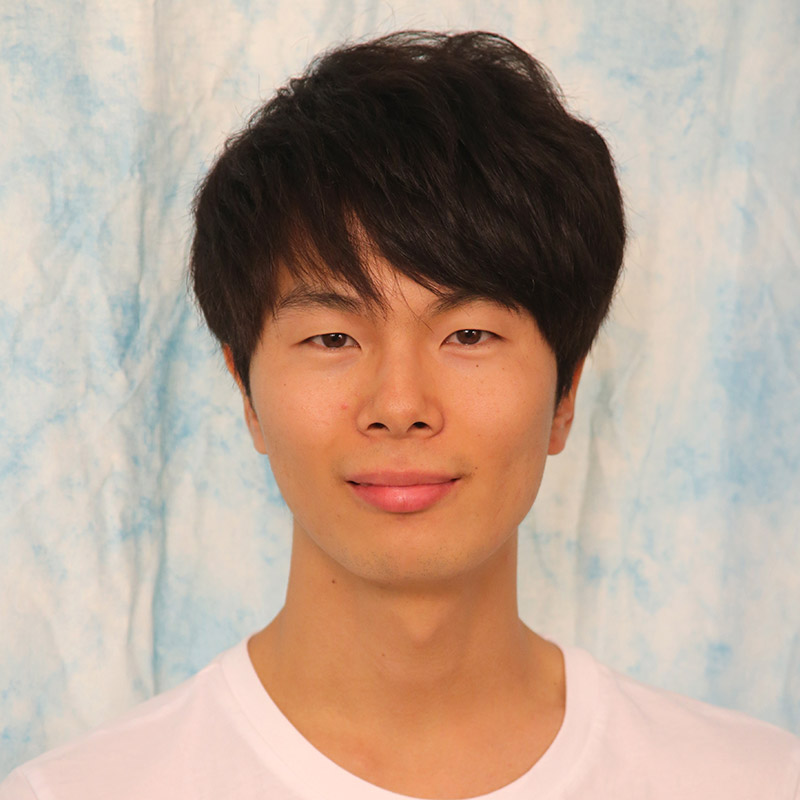
Carnegie Mellon University
2:00 pm to 3:00 pm
GHC 4405
Abstract:
Humans convey their thoughts, emotions, and intentions through a concert of social displays: voice, facial expressions, hand gestures, and body posture, collectively referred to as social signals. Despite advances in machine perception, machines are unable to discern the subtle and momentary nuances that carry so much of the information and context of human communication. The encoding of conveyed information by social signals, particularly in nonverbal communication, is still poorly understood, and thus it is unclear how to teach machines to use such social signals to make them collaborative partners rather than tools that we use. A major obstacle to scientific progress in this direction is the inability to sense and measure the wide spectrum of behavioral cues in groups of interacting individuals, which hinders applying computational methods to model and understand social signals.
In this thesis, we explore new approaches in sensing, measuring, and modeling social signals to ultimately endow machines with nonverbal communication abilities. This thesis starts by describing our exploration in building a massively multiview sensor system, the Panoptic Studio, that can capture the wide spectrum of human social signaling—including voice, social formations, facial expressions, hand gestures, and body postures—among groups of multiple people. Second, leveraging the more than 500 synchronized cameras, we then present a method to measure the subtle 3D movements of anatomical keypoints in face-to-face interactions, providing a new opportunity to computationally study social signals. In the last part of this thesis, we present a social signal prediction task to model nonverbal communication in a data-driven manner. We establish a new large-scale corpus from hundreds of participants containing various channels of social signal measurements. Leveraging this dataset, we verify that the social signals are predictive each other with strong correlations.
Thesis Committee Members:
Yaser Sheikh, Chair
Takeo Kanade
Louis-Philippe Morency
David Forsyth, University of Illinois Urbana-Champaign
Mina Cikara, Harvard University