
MSR Thesis Defense
July
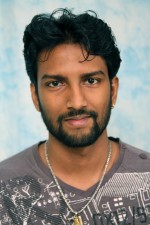
Carnegie Mellon University
12:00 pm to 1:00 pm
NSH 4513
Traditional methods for off-road terrain estimation use some type of learning network to predict hand labeled classes of terrain such as short grass, tall grass, dirt, and trees. Other methods of learning which can give more detailed, but stilldiscrete classes, use on board sensors to measure the terrain roughness, and then predict the terrain type. There also exists non-learning based approaches which use geometry and physics based equations along with inputs from sensors which give a measure of the terrain roughness or traversability. This thesis proposes to combine the best of both methods by developing two metrics for classifying roughness, an IMU based roughness metric and a point to plane distance roughness metric. Images auto-labeled images with these roughness metrics are then fed into a deep learning convolutional neural network designed for semantic segmentation. The result is a system which was trained without the subjectivity of a human, and with the ability
to predict roughness of outdoor terrain.
Committee Members:
George Kantor (Chair)
Kris Kitani
Sankalp Arora