
VASC Seminar
April
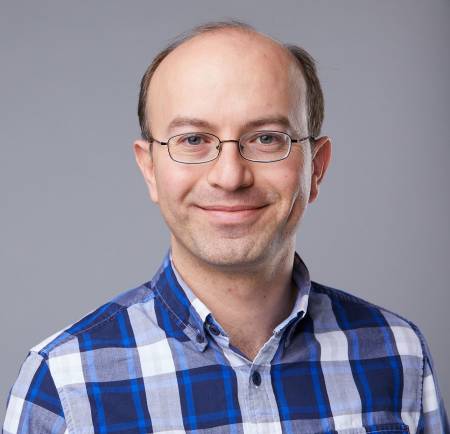
Abstract:
We are interested in learning visual representations that are discriminative for semantic image understanding tasks such as object classification, detection, and segmentation in images/videos. A common approach to obtain such features is to use supervised learning. However, this requires manual annotation of images, which is costly, ambiguous, and prone to errors. In contrast, self-supervised feature learning methods exploiting unlabeled data can be more scalable and flexible. I will present some of our recent efforts in this direction. More specifically, I will talk about our recent work on using similarity between a random set of images to learn better visual representations and also to compress self-supervised features from deeper models to smaller ones. Using ImageNet images only, our self-supervised AlexNet model outperforms the standard supervised AlexNet model on ImageNet task itself.
BIO:
Hamed Pirsiavash is an assistant professor at the University of Maryland Baltimore County (UMBC). He obtained his PhD at the University of California Irvine and did a postdoc at MIT. He does research in the intersection of computer vision and machine learning. More specifically, he is interested in self-supervised representation learning and also adversarial robustness of deep models.
Homepage: https://www.csee.umbc.edu/~hpirsiav/
Sponsored in part by: Facebook Reality Labs Pittsburgh