
PhD Speaking Qualifier
May
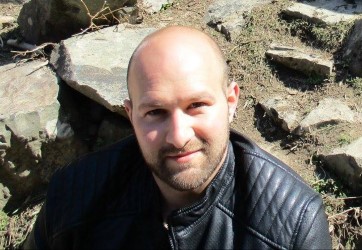
Carnegie Mellon University
3:30 pm to 4:30 pm
NSH 4305
Abstract:
For robots to operate robustly in the real world, they should be aware of their uncertainty, particularly when estimating the position and orientation, or pose, of objects. This uncertainty can be caused by many factors, such as occlusions, poor lighting, or object symmetry. These factors can naturally induce an inherent ambiguity in terms of the object’s pose, so a method for pose estimation should account for them by outputting a pose distribution. However, most methods for pose estimation output a single best guess of each object’s pose. In contrast, our method outputs a distribution over poses to take into account this ambiguity. We achieve this by learning a function that compares the image of the object to many different image of the object model, rendered at poses sampled uniformly from SO3. Each of these comparisons produces a score that, when normalized, yields a pose distribution. This distribution captures the uncertainty in our estimate of the object’s pose and can be used by downstream methods that rely pose estimation, such as grasping, motion planning, or object manipulation to determine the best actions to reduce or compensate for this uncertainty. We show results of our distribution estimation both on the YCB Video object poses estimation data set as well as a novel surgical instrument data set.
Committee:
Martial Hebert
David Held
Abhinav Gupta
Gunnar Sigurdsson