
MSR Thesis Defense
August
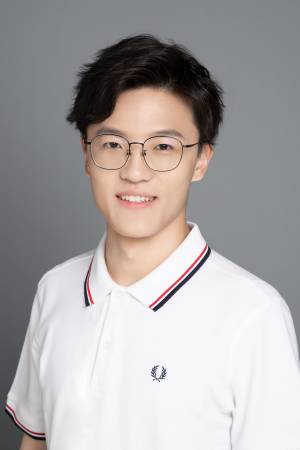
Title: Learning Legged Robot Agility: Sim-to-Real and Beyond
Abstract:
Legged robotics has seen significant advancements in both manipulation and locomotion. However, there remain significant gaps compared to their biological counterparts, particularly in energy efficiency, natural motion, and the capacity for agile skills. This thesis primarily focuses on two aspects: the unified control of legged manipulators and the development of novel control algorithms for multi-skill quadrupeds. The first study presents a strong counter to the standard hierarchical control pipeline for legged manipulators, which is characterized by immense engineering to support coordination between the arm and legs, often resulting in non-smooth unnatural motions. In this work, we propose to learn a unified policy for whole-body control of a legged manipulator using reinforcement learning. We propose Regularized Online Adaptation to bridge the sim2real gap for high-DoF control, and Advantage Mixing exploiting the causal dependency in the action space to overcome local minima during training the whole-body system. We also present a simple design for a low-cost legged manipulator, and find that our unified policy can demonstrate dynamic and agile behaviors across several task setups. The second study dives further into the field where robotic quadrupeds are still far behind their biological counterparts, such as dogs, which display a variety of agile skills and can use the legs beyond locomotion to perform several basic manipulation tasks like interacting with objects and climbing. We train quadruped robots not only to walk but also to use the front legs to climb walls, press buttons, and perform object interaction in the real world. To navigate this challenging optimization, we decouple the skill learning broadly into locomotion, involving movement whether via walking or climbing a wall, and manipulation, involving using one leg to interact while balancing on the other three legs. We also devise a behavior tree that encodes a high-level task hierarchy from one clean expert demonstration, thereby combining these skills into a robust long-term plan. Finally, we apply a sim2real variant that builds upon recent locomotion success to transfer these skills to the real world. Evaluations in both simulation and real-world settings exhibit successful executions of both short and long-range tasks, underscoring the robustness confronting external perturbations.
Committee:
Prof. Deepak Pathak (advisor)
Prof. Abhinav Gupta
Tianyi Zhang