
Special Talk
December
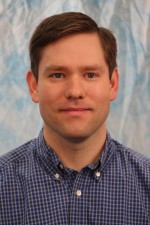
Abstract: Understandable-AI techniques facilitate to use of AI as a tool by human experts, giving humans insight into how AI decisions are made thereby helping experts discern which AI predictions should or shouldn’t be trusted. Understandable techniques may be especially useful for applications with insufficient validation data for regulatory approval, for which human experts must remain the final decision makers. One understandable-AI approach is to optimize a latent space representation to have specific human meaning, e.g. training each dimension of the latent space to represent a different human-labeled feature. A combination of learned classifiers and/or semantic segmentation systems could be trained to produce such a latent space. These latest-space features can optionally be refined with expert-provided heuristic functions. Relatively simple classifiers such as decision trees or tiny MLPs are a natural choice to infer predictions from such a (refined) latent space, since their classification logic can be easily traced by humans from inputs of understandable features to the output predictions. Recent causal discovery algorithms can yield simple MLP classifiers that potentially better model the physical realities underlying the data. Combined, human-understandable techniques such as these may make AI more trustworthy, facilitate knowledge discovery, and produce AI systems that teach the humans who are supervising the AI.