
MSR Thesis Defense
April
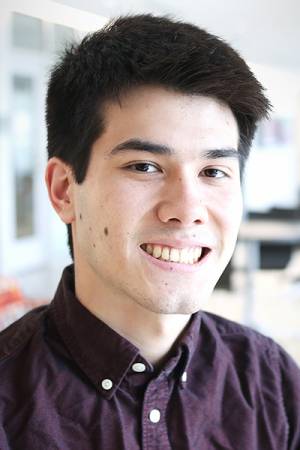
Abstract:
Off-road driving is an important instance of navigation in unstructured environments, which is a key robotics problem with many applications, such as exploration, agriculture, disaster response and defense. The key challenge in off-road driving is to be able to take in high dimensional, multi-modal sensing data and use it to make intelligent decisions on where and how to traverse over unstructured terrain. While off-road driving has been the focus of much research, it remains challenging to design systems that are capable of off-road driving quickly, robustly, and in a variety of environments.
In this thesis, we propose a learning-based system for off-road driving for a full-scale autonomous all-terrain vehicle. Key to the work in this thesis are two assertions: 1) we can design a high-performance system without any human-annotated data, and 2) learning should be used in concert with existing trajectory optimization methods for off-road driving. To address the first point, we collect a large dataset containing several hours of human-driven trajectories through challenging off-road terrain at high speeds. This dataset contains many traversability-stressing scenarios, such as steep slopes, dense vegetation and high speeds. To address the second point, we leverage this dataset to learn two key functions in trajectory optimization, the dynamics model and the cost function.
Overall, we find that our learning-based methods outperform traditional common-practice navigation baselines in isolation. More importantly, we also demonstrate that these learned models lead to improved performance when incorporated in path planning and control algorithms in large-scale, multi-kilometer navigation trials.
Committee:
Sebastian Scherer
Aaron Johnson
Cherie Ho