
VASC Seminar
February
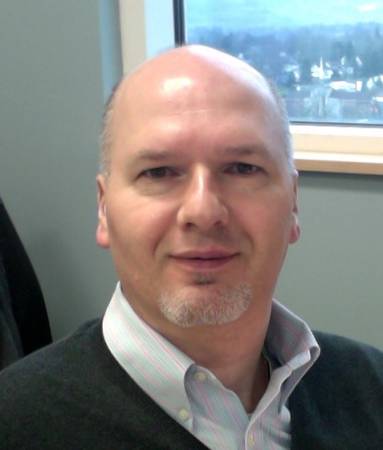
Abstract: Autoencoder neural networks are an unsupervised technique for learning representations, which have been used effectively in many data domains. While capable of generating data, autoencoders have been inferior to other models like Generative Adversarial Networks (GAN’s) in their ability to generate image data. We will describe a general autoencoder architecture that addresses this limitation, and that can learn effective representations while preserving the same generative properties of GAN’s. We will describe two applications of this architecture. The first one will demonstrate how autoencoders can learn several image manifolds even at high resolution (e.g., faces), allowing multiple image manipulations. The second will illustrate how to augment autoencoders for learning image manifolds for the purpose of detecting anomalies, or outlier data.
Bio: Gianfranco Doretto is an Associate Professor of Computer Science and Electrical Engineering at West Virginia University. His research interests span several computer vision and machine learning areas with a focus on developing deep learning methods for biometrics, robotics, digital health, and computational neuroscience applications. Gianfranco completed a D.Eng. in Electronics Engineering with highest honors at the University of Padua in 1998, and a Ph.D. in Computer Science at UCLA in 2005. After that, he was a Lead Scientist at GE Research for more than five years and was Visiting Professor at the University of Cagliari in 2012. Gianfranco directs the Vision and Learning Lab at WVU, and also co-founded IstoVisio Inc, the makers of syGlass, pioneering the era of large-scale biomedical data analysis and exploration in virtual reality.
Homepage: https://vision.csee.wvu.edu/~doretto/
Sponsored in part by: Facebook Reality Labs Pittsburgh