
PhD Speaking Qualifier
June
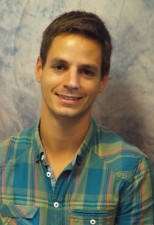
Carnegie Mellon University
4:00 pm to 5:00 pm
Abstract:
Obtaining large annotated datasets is critical for training successful machine learning models and it is frequently a bottleneck in practice. Weak supervision offers a promising alternative for producing labeled datasets without ground truth annotations by generating probabilistic labels using multiple noisy heuristics. This process can scale to large amounts of data and it has demonstrated state of the art performance in a range of diverse applications. One practical issue of learning from user-generated heuristics is that their creation requires creativity, foresight, and expertise from those who hand-craft them, a process which can be tedious and subjective. We develop the first framework for interactive weak supervision in which our method suggests heuristics and learns from feedback provided by experts. Our experiments demonstrate that only a small number of iterations are needed to train models that achieve highly competitive test set performance without access to ground truth training labels. We conduct experiments with real users which show that they are able to judge useful heuristics and that test set results track the performance of simulated oracles.
Committee:
Artur Dubrawski (advisor)
Oliver Kroemer
Jeff Schneider
Nick Gisolfi