
Field Robotics Center Seminar
January
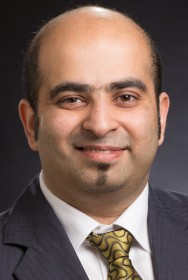
1:00 pm to 2:00 pm
Event Location: 1507
Bio: Girish Chowdhary received his PhD and MS degrees from Georgia
Institute of Technology. He has postdoctoral research experience at
the Georgia Tech UAV Research Facility, and at the Laboratory for
Information and Decision Systems and the School of Aeronautics and
Astronautics of the Massachusetts Institute of Technology. He also has
research experience at the German Aerospace Center’s (DLR’s) Institute
of Flight Systems. He is currently an assistant professor at Oklahoma
State University’s School of Mechanical and Aerospace Engineering.
Girish’s ongoing research interest is in creating provable algorithms
to enable intelligent adaptive autonomy for large-scale and
long-duration operation involving collaborating mobile agents. He has
authored over 50 peer reviewed papers and made several key
contributions to adaptive control, aircraft system identification,
fault tolerant control, distributed sensing and inference, Bayesian
nonparametric learning and control, optimal mission planning over long
durations, and vision aided navigation and control. His work has been
applied and demonstrated on several research UAVs and he has been
deeply involved in the development and automation of over 10 research
UAVs.
Abstract: Unmanned Aerial Vehicles (UAVs) are envisioned to bring the needed
capabilities in forward base support, disaster management, law
enforcement, and other grand challenge applications without putting
humans at direct risk. In order to realize this vision, UAVs must
learn to “fly themselves” and reliably. The key challenge in realizing
such technology arises from the need to adapt to uncertainties of
real-world operation, such as the lack of precise mathematical model
of the UAV and its operating environment, noisy measurements, changes
in configuration, lack of sensory aids such as GPS, mechanical damage,
and potential adversarial action. In this talk, I will begin by
presenting recent advances in information enabled adaptive control
methods designed to learn compact representations of the uncertainty
by concurrently making efficient use of all online available data,
including instantaneous measurements and online-recorded data. They
have formal guarantees that ensure desirable closed loop properties,
improved performance, and long-term learning. I will present how
online inference over Gaussian Process (GP) Bayesian Nonparametric
(BNP) models can lead to guaranteed stable adaptive controllers that
optimally learn the uncertainty in presence of stochasticity. I will
present results of several flight-test experiments that show how the
predictive variance of the GP can be used to guide exploration and
provides the adaptive controller with a metric to employ its learning
only when there is sufficient confidence in the learned model. In
addition, I will present an overview of our work in monocular vision
enabled control of rotorcraft UAVs in GPS denied environments.