
VASC Seminar
June
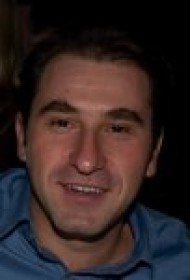
12:00 am to 12:00 am
Bio: Cristian Sminchisescu is a Professor in the Department of Mathematics, Faculty of Engineering, at Lund University. He has obtained a doctorate in computer science and applied mathematics with specialization in imagining, vision and robotics at INRIA, France, under an Eiffel excellence doctoral fellowship, and has done postdoctoral research in the Artificial intelligence Laboratory at the University of Toronto. He holds a Professor equivalent title at the Romanian Academy and a Professor rank, status appointment at Toronto, and conducts research at both institutions. During 2004-07, he has been a Faculty member at the Toyota Technological Institute, a philanthropically endowed computer science institute located at the University of Chicago, and during 2007-2012 on the Faculty of the Institute for Numerical Simulation in the Mathematics Department at Bonn University. He is a member of the Editorial Board (Associate Editor) of IEEE Transactions for Pattern Analysis and Machine Intelligence (PAMI). He has offered tutorials on 3d tracking, recognition and optimization at ICCV and CVPR, the Chicago Machine Learning Summer School, the AEFRAI Vision School in Barcelona, and the Computer Vision summer school at ETH in Zurich. Over time, his work has been funded by the United States National Science Foundation, the Romanian Science Foundation, the German Science Foundation, and the European Commission, under a Marie Curie Excellence Grant. Cristian Sminchisescu’s research goal is to train computers to `see’ and interact with the world seamlessly, as humans do. His research interests are in the area of computer vision (articulated objects, 3d reconstruction, segmentation, and object and action recognition) and machine learning (optimization and sampling algorithms, structured prediction, sparse approximations and kernel methods).
Abstract: I will give an overview our recent work on semantic visual interpretation based on image segmentation techniques. Differently from existing bag-of-words or regular-grid description methods that bypass image segmentation entirely, and unlike methods that segment images and recognize objects by detecting known object parts or fusing superpixel maps by means of random field models, we will explore interpretation strategies based on multiple figure-ground segmentations. Central to our approach is a recent combinatorial parametric max flow methodology (CPMC) that can explore, exactly, a large space of object layout hypotheses constrained at different image locations and spatial scales, in polynomial time. Once a potentially large ensemble of such hypotheses is obtained, we show that it is possible to distill and diversify a pool of a few hundred elements, at minimal loss of accuracy, by training category-independent models to predict how well each segment hypothesis exhibits real world regularities based on mid-level properties like boundary smoothness, Euler-number or convexity. I will show that such a simple combinatorial strategy operating on only low-level and mid-level features can generate segments that cover entire objects or parts in images with high probability and good accuracy, as empirically measured on most existing segmentation benchmarks. Moreover, the figure-ground segment pool can be now used within a sliding-segment- as opposed to sliding window – strategy, and in conjunction with second-order pooled region descriptions, for object detection, semantic segmentation or monocular 3d human pose reconstruction. A proof of concept system based on such principles has been demonstrated in the PASCAL VOC semantic segmentation challenge where it was top-ranked over the past four editions.
Joint work with J. Carreira, A. Ion, C. Ionescu, F. Li.