
VASC Seminar
November
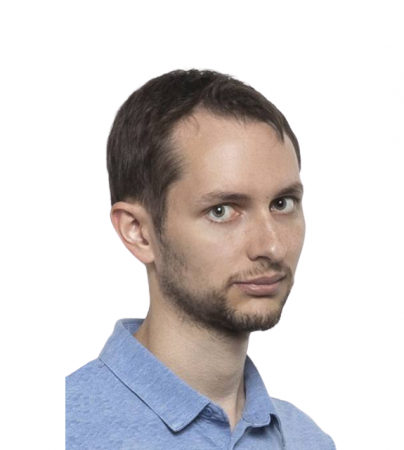
Abstract:
Linear Restoration Problems (or Linear Inverse Problems) involve reconstructing images or videos from noisy measurement vectors. Notable examples include denoising, inpainting, super-resolution, compressive sensing, deblurring and frame prediction. Often, multiple such tasks should be solved simultaneously, e.g., through Regularized Least Squares, where each individual problem is underdetermined (overcomplete) with infinitely many solutions from which a specific one should be selected. I show how joint end-to-end gradient-based self-supervised learning is possible for the Regularizer (defined implicitly via its proximal operator by a Deep Neural Network) and the Least Squares solver, giving rise to accurate and fast reconstruction algorithms that can be extended very easily. The presented work is a joint project with Barnabás Póczos from the Machine Learning Department of Carnegie Mellon University.
BIO:
Zoltán Ádám Milacski (31 years old) has a Bachelor’s degree in Mathematics and a Masters’ degree in Computer Science. He is currently a PhD Candidate awaiting his thesis defense in Machine Learning, focusing on the combination of Deep Neural Networks and Optimization Problems. He has published first author research papers at ICONIP’15, IJCNN’19, BMVC’19 and ICML’20 conferences, among several other co-authored works.
Homepage: https://scholar.google.com/citations?user=rSqodggAAAAJ
Sponsored in part by: Facebook Reality Labs Pittsburgh