
Field Robotics Center Seminar
May
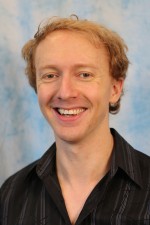
12:00 pm to 1:00 pm
NSH 1507
Abstract:
Unmanned aerial vehicles have many potential applications, such as monitoring crops and inspecting infrastructure. The potential benefits are greater if the UAV is semi- or fully-autonomous, requiring only occasional human oversight or none at all. This would allow the above use cases to be performed at lower cost, during any time of day, or enable new possibilities such as autonomous package delivery.
However, whilst aircraft generally stay out of urban areas, the possibility of UAVs operating in the lowest 50 feet of civilian airspace brings many technical challenges. We want to enable UAVs to operate very close-too, and possibly even interact with obstacles. Our general approach is to create a static map of the world and to plan the entire mission for the UAV. Our world model consists of a 3D Octree representation and a 2D height grid of the ground surface. We use a sample-based planning approach with rejection-sampling for constraint satisfaction. We also plan a complex roadmap of alternative paths so that if the vehicle encounters an obstacle during the mission, it only has to choose which obstacle-avoidance maneuver to execute. The map is high-resolution so the UAV can avoid trees or power lines in order to achieve the task, such as delivering a package to your front door. The UAV localizes in the static map using a LiDAR and VO-based odometry method. The benefits of our planning method are that it finds a path in less time than previous methods, such as Visibility Graphs (“SPARTAN”), with a comparable total path length. We can also plan in larger, higher-resolution maps than with previous methods, such as 3D Cost Maps.
This allows the UAV to fly through overhanging structures or into windows of derelict buildings. Our method also produces altitude-constrained paths and smooth take-off/landing motions. For collision-avoidance, we show faster decision making in cluttered spaces which leads to more safe vehicle behavior. For large, open spaces our method has similar performance to previous work using online planning with motion primitives.
Speaker Bio:
David Butterworth is an M.S. student in the Robotics Institute advised by Sanjiv Singh. He earned a B.Eng with honors in Mechatronics from the University of Queensland, and a Dip in Robotics and Mechatronics from Swinburne University in Melbourne, Australia. His current work focuses on motion planning for an autonomous aerial vehicle.