
VASC Seminar
February
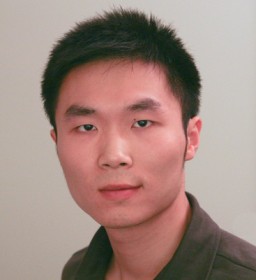
3:00 pm to 4:00 pm
Event Location: NSH 1507
Bio: Jia Deng is a Visiting Assistant Professor in the EECS department of the University of Michigan, where he will start as an Assistant Professor in Fall 2014. He received his Ph.D. from Princeton University in 2012 and his B.Eng. from Tsinghua University, both in computer science. He has been co-organizing the ImageNet Large Scale Visual Recognition Challenges since 2010. He is also the lead organizer of the BigVision workshops at NIPS 2012 and CVPR 2014. He won the ICCV Marr Prize in 2013 and his work has been featured in popular press such as the New York Times.
Abstract: Having machines recognize everything in our visual world is one of the grand challenges of computer vision. It entails building a system capable of distinguishing tens of thousands, if not millions, of fine-grained visual classes across a wide range of domains (e.g.distinguishing different breeds of terriers or different Toyota models). The advancement of visual recognition thus calls for approaches that address the large scale and complexity in both visual and semantic space.
In this talk I will present my research that takes a big data approach to scaling up recognition. I will start with the latest developments and discoveries of the ImageNet project, which harvests big visual data through large-scale crowdsourcing. Next, I will demonstrate how to recognize fine-grained, sub-ordinate categories via a gamification framework that leverages new forms of knowledge from the crowd. Third, I will explore ways to tackle the large label space, one with tens of thousands of visual categories organized in a large taxonomy. In particular, I will present techniques for building a reliable large-scale recognition engine. Finally, I will discuss future directions that hold promise for unleashing the full power of big data toward large-scale, real-world computer vision.